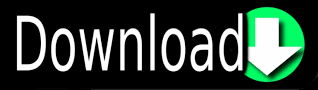
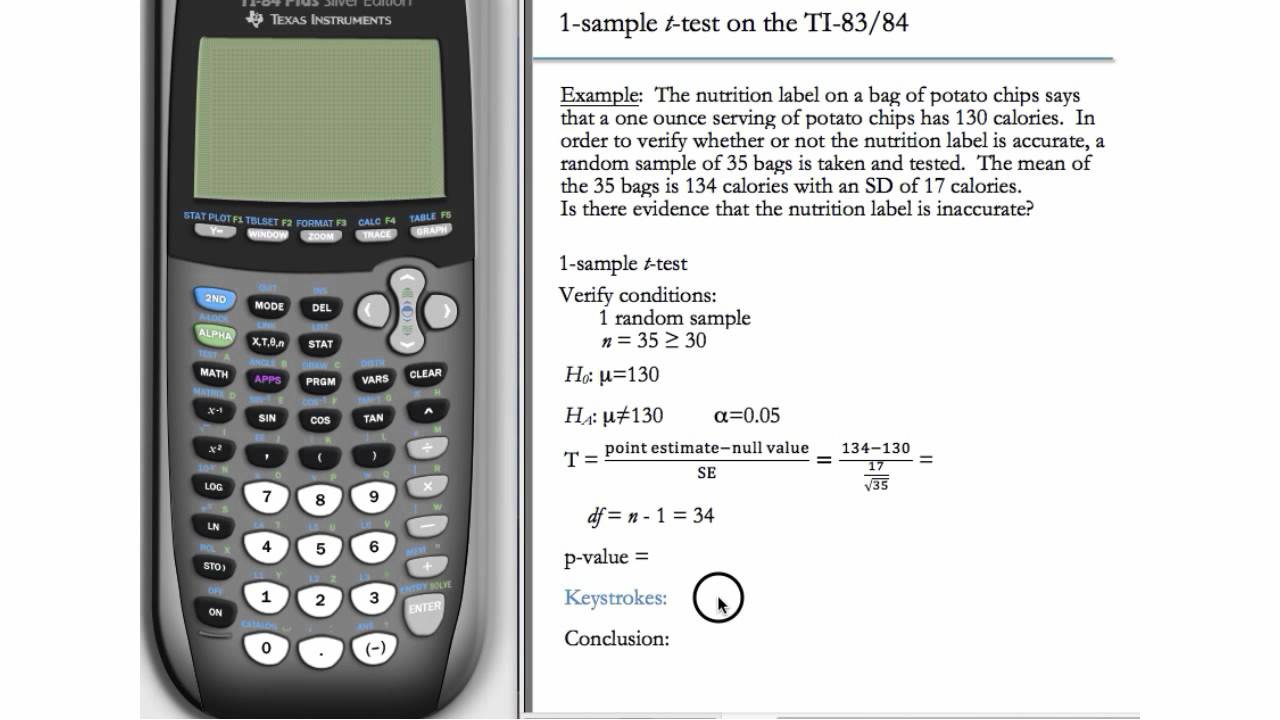
This is not the same as saying that the true mean equals the hypothetical value, but rather states that there is no evidence of a difference. If the p value is large (usually defined to mean greater than 0.05), the data do not give you any reason to conclude that the population mean differs from the designated value to which it has been compared. The p value answers this question: If the data were sampled from a Gaussian population with a mean equal to the hypothetical value you entered, what is the chance of randomly selecting N data points and finding a mean as far (or further) from the hypothetical value as observed here? The test produces a P value which requires careful interpretation. This hypothetical mean can be based upon a specific standard or other external prediction.
One sample t hypothesis test calculator how to#
How to interpret results of a one sample t testĪs discussed, a one sample t test compares the mean of a single column of numbers against a hypothetical mean.
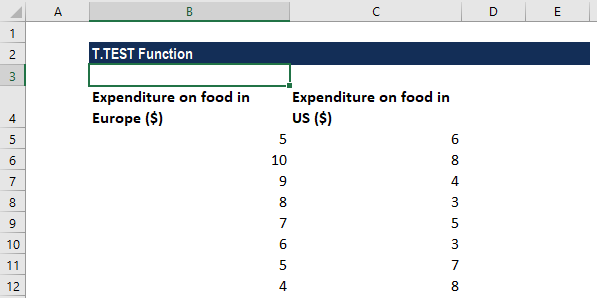
A final option is to use the t test anyway, knowing that the t test is fairly robust to departures from a Gaussian distribution with large samples. Another choice is to use the Wilcoxon signed rank nonparametric test instead of the t test. One option is to transform the values to make the distribution more Gaussian, perhaps by transforming all values to their reciprocals or logarithms. If your data do not come from a Gaussian distribution, there are three options to accommodate this. While this assumption is not as important with large samples, it is important with small sample sizes, especially less than 10. Normally distributed in the population (data should resemble a bell-shaped curve when plotted graphically)Īs with all parametric hypothesis testing, the one sample t test assumes that you have sampled your data from a population that follows a normal (or Gaussian) distribution. The results of a t test should be based on a random sample and only be generalized to the larger population from which samples were drawn. Obtained from a random sample of the population As they rely on the calculation of a mean value, variables that are categorical should not be analyzed using a t test. Prism cannot test this assumption, but there are graphical ways to explore data to verify this assumption is met.Ī t test is only appropriate to apply in situations where data represent variables that are continuous measurements. The results of a t test only make sense when the scatter is random - that whatever factor caused a value to be too high or too low affects only that one value. The term "error" refers to the difference between each value and the group mean. The one sample t test assumes that all "errors" in the data are independent. Independent (values are not related to one another): The null hypothesis for a one sample t test can be stated as: "The population mean equals the specified mean value." The alternative hypothesis for a one sample t test can be stated as: "The population mean is different from the specified mean value." Like all hypothesis testing, the one sample t test determines if there is enough evidence reject the null hypothesis (H0) in favor of an alternative hypothesis (H1). Often, this designated value is a mean previously established in a population, a standard value of interest, or a mean concluded from other studies. This designated value does not come from the data itself, but is an external value chosen for scientific reasons. The one sample t test, also referred to as a single sample t test, is a statistical hypothesis test used to determine whether the mean calculated from sample data collected from a single group is different from a designated value specified by the researcher. In this article you will learn the requirements and assumptions of a one sample t test, how to format and interpret the results of a one sample t test, and when to use different types of t tests.
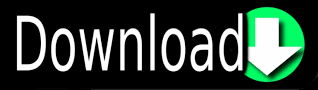